Researchers have recently demonstrated how an adaptive design strategy, based on comprehensive information, is able to accelerate the discovery of new materials with tailored properties.
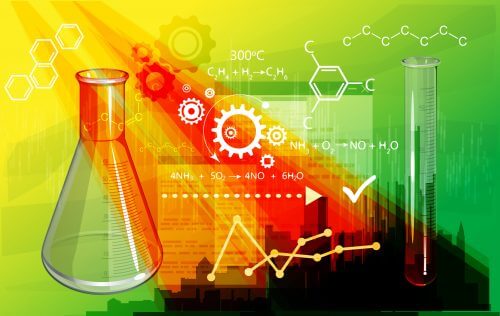
[Translation by Dr. Nachmani Moshe]
Researchers have recently demonstrated how an adaptive design strategy, based on comprehensive information, is able to accelerate the discovery of new materials with tailored properties. The research findings were recently published in the scientific journal Nature Communications.. "What we did was to prove that from a fairly limited collection of data from specially controlled experiments it is possible to direct the following experiments with the aim of finding the material with the required property," said Turab Lookman, a physicist at Los Alamos National Laboratory in the USA.
"Finding new materials was traditionally done by intuition and through 'trial and error'," explains the researcher. "However, with the rise in chemical complexity, the combination possibilities became too many within the framework of the 'trial and error' approach." In order to solve this problem, the researchers used machine learning to speed up the discovery process. The researchers were able to develop a framework that makes use of the uncertainties to guide subsequent experiments with the goal of finding memory alloys with very low thermal dissipation. Alloys of this type are essential to improve their longevity and stability in engineering applications. "Our goal was to halve the time and cost required to introduce new materials to the market," said the lead researcher. "We were able to demonstrate that a framework based on data and utilizing computational learning can lead to the discovery of new materials with required properties much faster than before." Most of the effort in the field of discovering new alloys has focused on the creation and scanning of databases accumulated following the execution of thousands of quantum mechanical calculations. However, the interrelationships between the structural, chemical and microstructural degrees of freedom cause high complexity.
The researchers focused on memory alloys based on nickel and titanium, however - the approach can be used for any group of materials (polymers, ceramic materials or nanomaterials) or for any required property (for example, electrical insulation, piezoelectric coefficients and the size of the gaps in conduction bands). The new method becomes important when the experiments or calculations are particularly expensive and/or take up too much time. Although the research focused on the world of chemistry, it can be easily adapted to processes consisting of many influencing factors.
Feedback from experiments: The process for obtaining data collection is increased with four new alloys. [Courtesy: Los Alamos National Laboratory]
One response
Machine learning is very common today. On the one hand, this is excellent mathematics - breaking the conventions of other mathematics. A non-deterministic adaptive algorithm - looks for a solution and finds it. In mathematics, we are used to deterministic proofs and abstract thinking.
On the other hand, many use it instead of physical knowledge to identify patterns in many fields. It seems that the natural selection in scientific works will filter machine learning works that are practically effective but without intuitive understanding, and between machine learning as a tool in the hands of researchers to provide additional research capacity different from what they previously knew as forms of thinking - cluster theory, probability, logical proofs, fuzzy logic.