Since learning from examples requires a lot of computing power, it is often performed on computers that contain graphics processors (GPUs) that excel at this. However, the speed of these processors is still relatively low compared to the desired learning rate of the neural networks
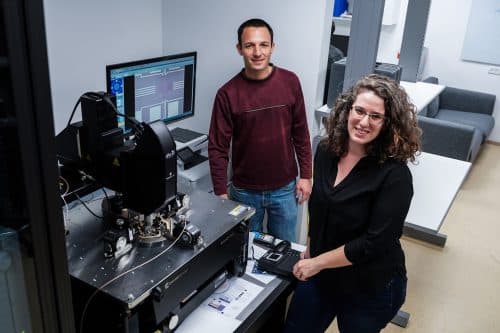
Researchers at the Viterbi Faculty of Electrical Engineering at the Technion have developed innovative hardware that accelerates the learning process of artificial intelligence systems. The research was led by Dr. Shahar Kutinsky and doctoral student Tsefnat Greenberg-Toledo, and students Roi Mazor and Amir Haj Ali participated in it. Their article was published in the journal IEEE Transactions on Circuits and Systems published by the International Society of Electrical and Electronics Engineers (IEEE).
In recent years, significant progress has been made in the world of artificial intelligence, mainly thanks to deep neural networks (DNNs) models. These networks, which were designed inspired by the human brain and human learning methods, perform with unprecedented success complex tasks such as autonomous driving, natural language processing, recognizing emotions in text, translation, image recognition and developing innovative medical treatments. This is through self-learning from a huge pool of examples - pictures, for example. This technology is developing rapidly in academic research groups as well as in huge companies such as Facebook and Google, which harness it for their needs.
Since learning from examples requires a lot of computing power, it is often performed on computers that contain graphics processors (GPUs) that excel at this. However, the speed of these processors is still relatively low relative to the desired learning rate of the neural networks, so the processor is still a bottleneck in this process. Furthermore, the use of processors consumes a lot of energy. According to Dr. Kotinsky, "we actually have hardware here that was originally intended for other uses - graphics, mainly - and it does not keep up with the fast pace of the activity that occurs in the neural networks. To solve this problem we need dedicated hardware that is adapted to work with deep neural networks."
Indeed, Dr. Kotinsky's research group has developed, on a theoretical level, hardware systems that are specially adapted to work with these networks and allow the neural network to perform the learning phase at high speed and with reduced energy. According to Dr. Kutinsky, "compared to working with graphics processors, our hardware improves the calculation speed 1,000 times and reduces energy consumption by 80%."
The hardware developed by the group constitutes a real breakthrough and conceptual change: instead of improving existing processors, the Technion researchers developed a structure for a XNUMXD computing machine that integrates memory. "Instead of splitting between the units that perform the calculations and the memory responsible for saving the information, we do everything inside the meristor - a memory component with computational power that is used in this case specifically for working with deep neural networks."
Although this is a theoretical work, the group has already demonstrated the application of the development at the simulation level. According to Dr. Kotinsky, "Our development is designed to work with the 'Momentum' learning algorithm, but the intention is to continue developing the hardware so that it is also compatible with other algorithms. It is possible that instead of several different hardware components, we will develop dynamic, multi-purpose hardware that can adapt itself to different algorithms.
4 תגובות
There are quite a few startups, including Israeli ones, that develop dedicated hardware for deep learning networks. They already have hardware in hand. They also talk about similar numbers of improving calculation speed and energy consumption. So what's new? I'm almost certain that the guys from the Technion know about this, so if you're going to post on the science website, then let it be at the proper level of depth...
Well done. There are studies and there are studies. There are developments and there are developments. This system is at the highest level.
Google has the TPU platform for fast calculations on tensors (in its third generation now). Is the development at the Technion better than this?
Breathe