Two spouses from the USA who are researchers at the Vitzin Institute: Yakir Rashef and Hilary Pinoken jointly signed an article published recently in the journal Science, which deals with relationships
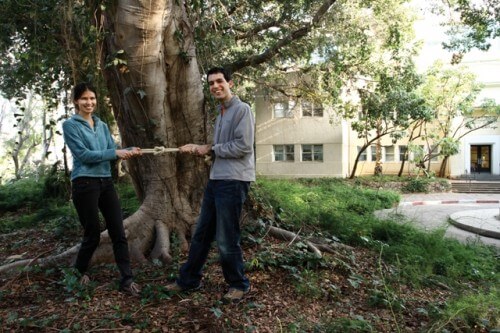
Connections are a complicated matter, but fortunately for Yakir Rashef, it seems that discovering significant connections is actually his strong point. Yakir, who was born in Israel in 1987 and grew up in the USA, met Hilary Finnoken in middle school, and since then the relationship between them is growing stronger: both studied in the mathematics department at Harvard University, and both are currently in the Faculty of Mathematics and Computer Science at the Weizmann Institute of Science. Yakir is a researcher supported by a Fulbright scholarship, as a guest student in the group of Prof. Moni Naor, and Hilary is studying for a PhD in the group of Prof. Itai Binyamini.
In light of all this, it should not be surprising that the two couples jointly signed an article published recently in the journal Science, which deals with relationships. What may be surprising, however, is that another partner in the article is Yakir's brother, Dr. David Rashef, a scientist in the field of computer science at the Broad Institute next to the Massachusetts Institute of Technology and Harvard University (Broad Institute of MIT and Harvard). The article presents a new method for processing information, which is capable of scanning complex sets of data, and locating interesting relationships and trends - which cannot be identified by other means of statistical analysis.
"When I was a student at Harvard, my brother asked me to help him create a computer program that would visualize and analyze large data sets in the field of public health. When we started working, we discovered that in order to do this, you must first decide which connections to take into account", explains Yakir. This condition, which may sound simple, gets more complicated as the data sets grow. Thus, for example, microbiologists who are interested in analyzing relationships between bacterial populations residing in the intestines of humans and other mammals are faced with trillions of bacteria. Even if we reduce the data set to contain only 7,000 bacteria, we still get over 22 million possible connections between pairs of bacteria. It is a vast ocean of information, as long as we don't know what types of patterns to look for. Challenges of this type, which include data sets that are based on thousands of variables, are becoming more common in various fields such as genomics, physics, political science, economics, and more, and the demand for efficient tools for processing the information is growing.
The scientists realized that they needed an algorithm that could discover new and important connections, but also unexpected ones - ones that might have escaped the eye. The method they developed - under the guidance of Prof. Michael Mitzenmacher from the School of Engineering and Applied Sciences at Harvard, and Prof. Pardis Sabati from the Broad Institute - is called "Maximal Information Coefficient" (MIC). It is based on the idea that if there is a relationship between two variables, it is possible to determine value bars for each of them - which together will create a common grid - which will highlight the relationship. The algorithm that calculates the maximum information coefficient scans the many lattices that can be created in this way, chooses the best among them, and quantifies the strength of the connection based on it. It is possible to calculate the maximum information coefficient for each pair of variables in the data set, rank the pairs according to the score they received (the higher the score, the stronger the relationship), and then examine the pairs that received the highest score - that is, the variables with the strongest influence most on each other.
To test the new method, the scientists applied it to a number of data sets, in areas such as public health, gene expression, intestinal bacterial populations, and baseball leagues, and compared the results of the new algorithm to the results obtained by other methods.
In analyzing the gut bacteria data, the algorithm was able to reduce the 22 million pairs of variables to several hundreds of interesting relationships, many of which were not discovered using other methods. Thus, for example, situations of "non-existence at the same time" were discovered, that is, when one type of bacteria is very common, another type is not common. Some of those situations of "non-existence at the same time" are known cases, and it is known that they are caused by the food consumed by the animal in which the bacteria reside, while other situations were unique, and hinted at the possibility that there is another factor, apart from the type of food, which affects this situation.
A graph depicting the relationship between different subspecies of intestinal bacteria
A graph depicting the relationship between different subspecies of intestinal bacteria. The nodes represent the subspecies, and the edges connecting them represent the 300 main non-linear relationships. The size of the node is proportional to the number of its connections. Black bars represent relationships explained by food intake. The nodes are surrounded by a color according to the relative part of the black ribs, out of all the ribs adjacent to them (0% in blue, 100% in red)
In another example, the team of scientists examined a database of the World Health Organization, which includes 357 variables in 200 countries. One of the interesting connections, found in the Pacific Islands, was a direct relationship between obesity among women and the level of household income - unlike what happens in other countries, where obesity first increases and then decreases. A possible explanation for the unusual findings is that in these islands obesity is considered a status symbol. Many accepted methods will define such an abnormal trend as "background noise", but the new algorithm is able to identify the existence of connections even when it comes to different - and even opposing - trends.
The analysis of baseball data using the algorithm showed that the number of hits, the number of bases, and the number of innings produced by the player for the team are the main factors that determine his salary, while other statistical methods placed three other factors at the top of the list. Who is right? The researchers intend to let baseball fans decide what factors affect - or should affect - the players' salaries.
"Unlike other methods, our method gives a high score to a wide range of types of relationships hidden in large databases, but it is also able to give the same score to relationships hidden by background noise," says Hilary Pinoken. And Yakir Reshef adds: "In other words, it is able to find interesting things that you did not expect to find, and it is difficult to discover them with other analysis methods."
And as for Hilary and Likir, it seems that working together on the algorithm helped them define the type of relationship with the highest score for them - marriage. "It's really wonderful for us that we both share the love of mathematics", say the couple, who share other common loves - playing the piano, running and cooking.
3 תגובות
Is it even possible to prove that there are no connections between things?
It doesn't seem to me that the term knot that is used in statistical mathematical analyzes like in the article is related to the term real knot. A real connection is only a physical connection! It is true that a statistical correlation can speculate on the existence of a physical connection, but there is no proof of this, and it is certainly not possible to prove a real lack of connection.
* If
Very nice!
It would be nice if you would give links and more information about things written in the article; For the article in question for example.