A team of researchers from the Massachusetts Institute of Technology (MIT) are using artificial intelligence to accelerate the discovery of an intriguing phenomenon in special materials, a discovery that could lead to the development of electronic components that operate without energy loss
[Translation by Dr. Moshe Nachmani]
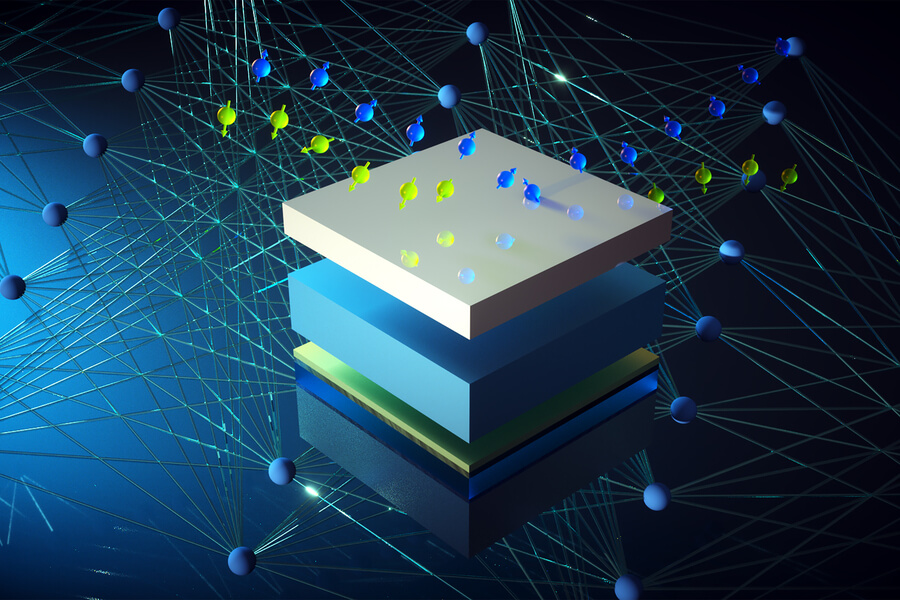
Superconductors have long been considered the primary approach to resistless electron transfer. In the last decade, a new family of quantum materials 'topological materials' has been developed which provides a promising alternative for the development of electronic components that operate without energy losses. Compared to superconductors, topological materials have several advantages, such as resistance to interference. In the field of magnetism, the phenomenon of "magnetic proximity effect" (magnetic proximity effect) is known, which occurs when the magnetism penetrates somewhat into the surface of a topological material. At the same time, watching this result has so far been particularly challenging. Explains researcher Zhantao Chen from the Massachusetts Institute of Technology (MIT: "The presence of this effect is usually too weak to be measured using existing methods."
During the last few years, researchers have relied on measuring this result on a method known as "polarized neutron reflectometry" (polarized neutron reflectometry) which allows testing of the deep magnetic structure of multi-layered materials. Within this method, two polarized neutron beams with opposite spin are returned from the sample and reach the detector. "If the neutron hits a magnetic flux of opposite direction, such as the one inside a magnetic material, it will change its spin state, which will lead to the measurement of different signals from the two neutron beams with up/down spin", explains one of the researchers. As a result, the proximity effect can be measured if a thin layer of intrinsically non-magnetic material - located near the magnetic material - becomes magnetic. However, the result is very subtle, reaching the level of only one nanometer in depth, and its measurement becomes challenging and inconclusive when it is disturbed by the results of the experiment itself. "By incorporating 'machine learning' into our method, we hope to get a clear picture of the mechanism," explains the lead researcher. This hope was indeed realized when the findings of the research team were recently published in the prestigious scientific journal Applied Physics Review.
The researchers examined a topological insulator - a material that insulates electricity in its inner part, but is able to conduct an electric current on its outer surface. They chose to focus on layered materials containing the topological insulator bismuth selenide (Bi2Se3) combined with the ferromagnetic insulator europium sulfide (EuS). Bismuth selenide, in essence, is a non-magnetic material, so the europium sulfide layer is responsible for the difference between the signals measured by the two polarized neutron beams. In addition, with the help of machine learning, the researchers were able to identify and quantify another contribution to the PNR signal: the induced magnetism created at the interface between the two bismuth selenide/ europium sulfate materials. "Methods of machine learning are very effective in identifying the basis of complex data, and make it possible to distinguish and differentiate between subtle results such as proximity magnetism in PNR measurements," explains the researcher.
When the signal is first fed into a machine learning model it is very complex. The model is able to simplify this signal so that the proximity result is increased and becomes much more visible. In the next step, the model is able to quantify the induced magnetism - while determining if the result of the magnetic proximity is indeed observable - along with other properties of the material system, such as: thickness, density and hardness of the different layers. "We have reduced the lack of clarity that was obtained in previous tests, thanks to the doubling of the separation capacity resulting from the use of machine learning," explains the researcher. The significance of the results is that the researchers could distinguish the properties of the materials at a length of 0.5 nanometers, about half the typical spatial dimension of the proximity result. This situation is analogous to a person looking at the inscription on a blackboard about six meters away from him so that he cannot distinguish the separate words written there. However, if we halve that distance, we might be able to read the separate words. The data analysis process can also be significantly accelerated by relying on machine learning. "In the old days, the researchers were required to devote entire weeks to analyzing all the data until obtaining an imaging curve that would match the curve of the experimental results," explains the chief researcher. "A large amount of experiments is required since the signal itself can correspond to different combinations of indicators. The neural network provides you with an answer immediately. There are no more guesses - no more trial and error."
External parties have praised the new study - which is the first of its kind to measure the effectiveness of machine learning in identifying the proximity result, and among the first in general to analyze PNR data. "The research provides an alternative way to identify the meticulous details in PNR data, and shows how a higher separation capacity can be continuously achieved", explains one of the professors in the field.
The research group is already planning to expand the scope of its experiments. "The magnetic proximity result is not the only weak result that interests researchers today," explains the researcher. "The machine learning infrastructure we developed can be easily adapted to different types of problems, such as the proximity result of superconductors, which is one of the most important problems today in the field of quantum computing."
More of the topic in Hayadan:
- A new method to control the magnetic properties of graphene
- Israeli researchers manage to predict solar storms days in advance thanks to artificial intelligence
- Researchers are expanding the use of deep learning to distinguish ciphertext from random noise
- The movement of the tectonic plates works according to a chain reaction
- Evidence of agricultural and industrial activity was uncovered in Ramat Hasharon as early as 1500 years ago