The development, based on artificial intelligence, will make it possible to improve breast cancer treatments and warn of wrong diagnoses
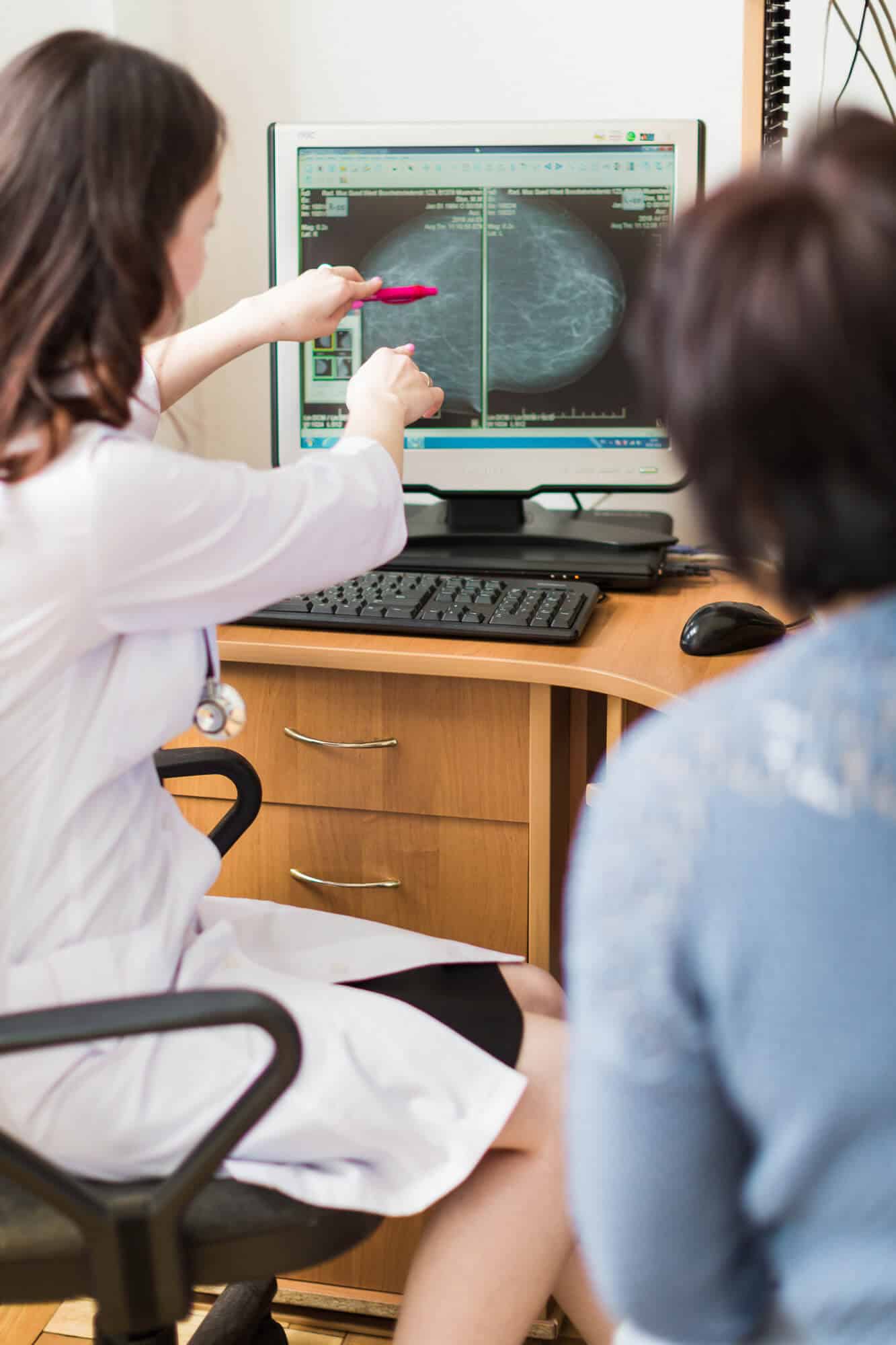
Computational models developed by researchers at the Taub Faculty of Computer Science will make it possible to better evaluate the effectiveness of immunotherapy treatments, predict the patient's life expectancy, improve treatments in a personalized way and warn of wrong diagnoses. The research on the subject is led by Dr. Gil Shamai, a post-doctoral student in the Laboratory for Geometric Image Processing (GIP) led by Prof. Ron Kimmel. Prof. Kimmel is a faculty member in the Taub Faculty of Computer Science and a professor with a secondary affiliation in the Viterbi Faculty of Electrical and Computer Engineering.
the article Which was published Now in the prestigious journal Nature Communications. Describes a method based on technology and experiments developed by Dr. Shamai with Amir Livna, a master's degree graduate from the laboratory, for the purpose of better evaluating the effectiveness of immunotherapy treatment in breast cancer. The researchers worked closely with Prof. Edmond Sebo, Director of the Institute of Pathology at Carmel Hospital; Dr. Alexandra Kerzo, a pathology specialist at the Carmel Hospital; and Dr. Antonio Polonia from the Ipatimup Pathology Laboratory in Portugal; And with the support of Prof. Gil Bar Sela, director of the oncology department at the Emek Hospital.
Breast cancer has become the leading cause of death among women aged 59-20 and the most diagnosed cancer in 2021, with approximately 12% of all diagnoses worldwide. The incidence of breast cancer in the population is increasing due to population growth, environmental pollution, industrialized nutrition, climate change and more. The immune system is able, in many cases, to eliminate cancer cells in a targeted and more effective way than drug therapy. The problem is that the cancer cells, which also developed in a long evolution, may develop mechanisms that prevent the immune system from attacking them. One of the innovative and experimental methods for treating breast cancer, in light of the success in treating other types of cancer, is immunotherapy - an approach that helps the immune system attack the cancer cells by damaging these mechanisms.
One of the common immunotherapy treatments is based on the interaction of a protein called PD-L1 with immune system cells. The interaction inhibits the immune system from working, and immunotherapy may prevent this interaction and thus help the immune system attack the tumor. However, the treatment is effective and approved for use only when PD-L1 proteins are expressed in the cancer cells themselves. Against this background, the need arose to differentiate between tumors characterized by the presence of the PD-L1 protein, in which immunotherapy treatment is expected to help, and tumors that are not. The problem is that measuring PD-L1 is not a simple task, and current measurement methods are expensive and imprecise.
"Unfortunately, I personally experienced the problematic nature of the existing methods during my father's anti-cancer treatments," says Prof. Kimmel. "These are inconsistent methods, that is - different measurements of the same phenomenon give different results. In addition, they are very expensive and not available everywhere, and the result is that the treatments are still given in a process that involves a lot of trial and error, meaning a huge waste of time and money and of course unnecessary suffering."
The research group developed following a request from an intern (in medicine) who heard about the wonders of artificial intelligence and turned to Prof. Kimmel and Dr. Shammai with the question: Can artificial intelligence help in the analysis of samples (biopsy) from breast cancer for the purpose of measuring the expression of receptors and proteins? "His proposal sounded very interesting to me and I started researching the issue," says Dr. Shamai. "I went to expert pathologists and they explained to me that the resolution of the tissue diagnosis is not sufficient to see receptors and proteins without special molecular staining. One of them even said it was like looking at a closed door and guessing what was in the room. This project became my research in the following years, because I wanted to prove them wrong." The research group was established a few years ago and currently employs a large group of engineers, clinicians and undergraduate, graduate and postgraduate students.
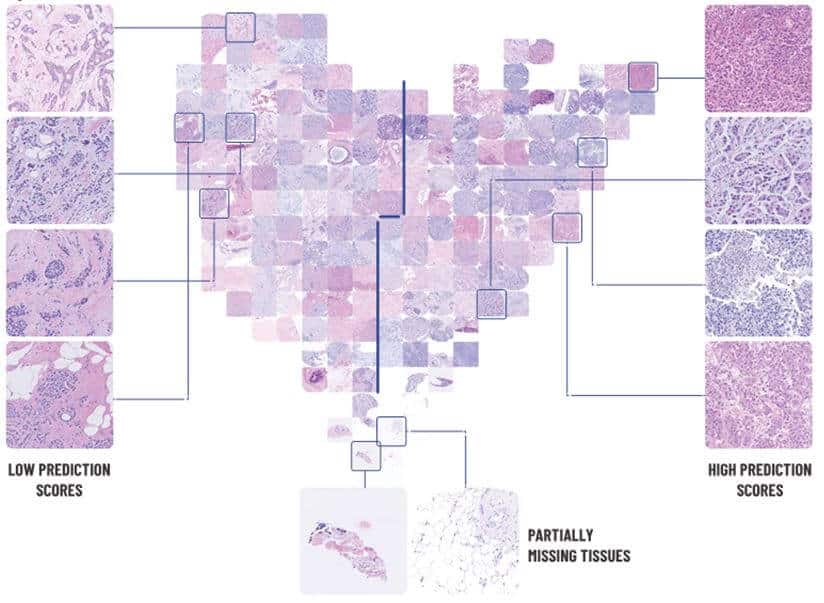
"Pathologists we spoke with said that this is an impossible task," explains Prof. Kimmel, "because a human pathologist cannot deduce the properties of the tumor from its shape due to the huge amount of variables. The good news is that artificial intelligence technologies and especially deep learning are able to do this. The computer, unlike the pathologist, and even the most skilled pathologist, can characterize the cancer with the help of a complex analysis of its morphology."
The first breakthrough in the research occurred about three years ago and was presented in the article in writing the time JAMA In which the group presented success in identifying receptors on cancer cells. The technology presented by the researchers is based on deep learning. It extracts molecular information from biopsy images that have undergone hematoxylin and eosin (H&E) staining - a common staining used to examine tissue taken in a biopsy. The staining allows the pathologist to identify in the tissue, under the microscope, the type of cancer and its degree of severity. However, the staining alone does not allow identifying proteins that are essential in determining the appropriate treatment. This is the challenge that the Technion researchers had to face.
Now, three years after the JAMA article, the researchers present success in measuring PD-L1 protein expression on a large sample pool: biopsies from more than 3,000 breast cancer patients. According to Dr. Shamai and Prof. Kimmel, "Deep learning requires a huge amount of information, and obtaining the required type of information is not easy. For this purpose, we wrote software code to scan online sources and automatically download thousands of biopsy samples and the relevant medical information approved for research use. The help of the pathologists who signed the study was very essential."
The computational model developed by the researchers does not "see the receptors and proteins" directly but knows how to infer their presence or absence based on the morphology of the tissue, or the "signature" that the cancer leaves in the tissue, as reflected in H&E scans. "This is such a complex relationship," says Dr. Shamai, "that the algorithm we developed knows how to provide us with the necessary information, but we don't really understand how it does it."
The research is based on advanced technologies of geometric processing of images - one of the research focuses in the laboratory of Prof. Ron Kimmel, in which Dr. Shamai's group operates. "It's a huge and complex challenge - producing a reliable and accurate clinical picture based on partial information," says Prof. Kimmel. "There are a lot of factors involved here - engineers, clinicians, students and ten hospitals in Israel and abroad. The research group was required to study the clinical aspects of breast cancer and its diagnosis and to create a huge database and tools to analyze the data and create a reliable prediction. Gil also had an enormous administrative task here, and he met it with impressive success, including obtaining seven research grants from foundations that recognized the importance of the project. In fact, he founded a kind of medical-technological startup here that presents dramatic achievements in the field of cancer."
The new technology has been verified (validated) on data from 300 breast cancer patients from a clinical trial. The researchers estimate that this approach, the analysis of pathological images using artificial intelligence, will become a significant factor in cancer research, in improving diagnosis and improving the appropriateness of treatment for the patient.
The research was supported by the Innovation Authority (Kamin grant) and by the National Science Foundation (the research program in personalized medicine).
for the article in the journalNature Communications. click here
More of the topic in Hayadan:
- Artificial intelligence in the service of cancer medicine
- Technology from the field of computational geometry developed at the Technion enables the production of unique inhalation masks for babies
- A witty award for Professor Shlomo Shamai
- Shlomo Havlin from Bar Ilan, Eli Keshet and Avner de Shalit from the Hebrew University and Shlomo Shamai from the Technion won the Rothschild Prize
- Cancer treatment: can a blood test replace an invasive procedure?
One response
"The incidence of breast cancer in the population is increasing due to population growth..." - it is not appropriate for the Technion to write such a thing.