Despite the lessons of the 2008 global credit crisis, Wall Street is betting on our future based on shaky scientific models
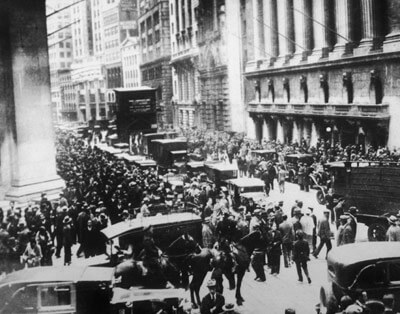
Many factors led to the collapse of the American financial market in 2008, which degenerated the entire world into an economic depression from which it has not yet been able to fully recover. One of the reasons for this is mathematics. Financial investment companies have developed such complex methods for investing their clients' money that, in order to assess the risks inherent in these investments, they had to rely on formulas that only connoisseurs can understand. However, as we learned from our bitter experience three years ago, these formulas, or models, are nothing but pale reflections of the real world, and at times they can mislead us and cause irreparable damage.
As we know, the financial field is not the only field where decisions are made based on mathematical models that cannot always be trusted. Scientists deal with models in many fields, such as climate science, beach erosion and nuclear safety, where either the phenomena the model describes are extremely complex, or it is difficult to obtain information about them, or both, as indeed happens in financial models. However, in no area of human activity are models founded on such a shaky foundation given so much credence as in the financial field.
It is the supposed power of the risk assessment models that gave the investment companies the confidence to use huge loans as financial leverage in order to increase the profits from their bets. These models were used by them to predict the degree of risk in these bets and to locate other investments that were supposed to offset this risk. But in view of the enormous uncertainties of the models in which the investment companies put their trust, it was nothing but false security. "We simply don't know enough to create a good theory that will be able to encompass all the financial risks we are exposed to," says David Kolander, an economist from Middlebury College, who studied the global credit crisis of 2008. "The idea is that we have models that are able to describe all the uncertainty and behavior The unpredictability we are witnessing in the markets is nothing but a crazy idea. But use these models as if they are actually capable of doing so.”
It would be too simplistic to blame the economic disaster on risk assessment models alone. Other human factors, political and regulatory, also undoubtedly played a role in the crisis. However, it is certainly possible to argue that the models were a critical link in the chain of events that led to the landslide, and perhaps even a decisive factor without which the economic disaster would not have occurred. In this situation, where so much is at stake, the financial investment companies in the last three years since 2008 have allocated tens of millions of dollars to strengthen their models for assessing investment risks, in the hope that the new models will prevent another economic collapse. But their hope may be false hope or baseless wishful thinking. Financial modeling experts express great doubt as to the possibility of any substantial improvement in risk assessment models. The meaning of this is both clear and frightening: banks and investment companies are leading the global economy into a future where there is a high risk of repeating the events of the past.
The pink future of the 2007 era
In a way, it is not difficult to understand the failure of risk assessment models in 2007 and 2008. These models were supposed to simulate the complex interactions between many market forces, such as market fluctuations, changes in interest rates, and the prices of stocks, bonds, options, and other financial instruments. Even if they succeeded in doing so, and this is debatable, the models did not take into account one important scenario: what happens when all the investors in the market want to sell all their holdings at the same time?
This is exactly what happened in those dark days of September 2008, when the American government decided not to rescue the investment bank Lehman Brothers from the economic crisis it had fallen into, and the respected financial institution was unable to pay its debts. Only a massive infusion of funds into the market by the US federal government stopped the snowball and prevented a total collapse.
During 2007, the risk assessment models predicted that the chances of any major financial institution going into default were minimal. According to Marco Avellaneda, a mathematician from New York University and an expert in financial risk assessment models, one of the most serious problems was that the models ignored a very important variable that affects the robustness of an investment portfolio: liquidity, that is, the ability of the market to coordinate the amount of buyers and the amount of sellers. When a key variable is not taken into account, it has considerable consequences.
An equation for predicting the risk of a delay in the landing time of an airplane will not be reliable if it does not include a mathematical factor that represents delays due to weather conditions. In our case, the liquidity factor is probably the most important variable in assessing the risk of insolvency in the case of mortgage-backed securities in all their versions. These securities are the complex financial instruments that were involved in the previous decade in the dizzying growth of the US housing market, and in particular in the sub-prime mortgages (loans with a high risk of not being repaid). When in 2008 housing prices in the US began to fall, no one knew how to truly estimate the value of these financial instruments, and this caused trading in them to stop, that is, they became "illiquid".
As a result, the banks that held them were left unable to pay off their debts, and this caused panic among investors. According to Avlanda, if the financial models had properly identified the risk of illiquidity, this would have allowed banks to lower the price of these financial instruments at an earlier stage and thus allow buyers to risk less money.
Ignoring a key factor seems like a scandal, but scientists do it all the time. Sometimes they are simply not aware that a certain variable plays a key role and sometimes they don't know how to take it into account in the equation. According to Kolander, this poses a problem in climate science, whose models often lack factors that represent the influence of clouds. "Among the factors that determine what the weather will be, clouds have a weight of 60%, and the models usually ignore them," says Kolander.
"When you cannot take into account in the model a factor that has such a significant effect on the results, you must exercise great judgment regarding the results, and not believe them blindly." This problem also arises in many other situations. How can the public's willingness to be vaccinated be expressed, when building a model for the spread of a new and dangerous type of flu? And what about modeling the ability of emergency teams to replace damaged parts and extinguish fires in nuclear power plants in the event of an overheating of the reactor core?
When we clearly identify an essential factor that we did not take into account in the model, for the most part, the hard way, it is sometimes possible to solve the problem, but sometimes it is not possible to fix what needs to be fixed. In the case of models for predicting financial risks, including the illiquidity factor in the model is not a trivial matter, since illiquidity tends to behave in a much more non-linear way than is customary in the case of prices, says Robert Jarrow, a professor of finance and economics at Cornell University whose work focuses on in risk assessment models. Markets can go from highly liquid to illiquid in the blink of an eye. This is similar to the difference between modeling the air flow around an airplane flying at normal speeds and modeling the flow created around an airplane exceeding the speed of sound (many airplanes ran into problematic situations before aviation engineers were able to build a model that would describe such a situation properly).
Jaro strives to incorporate the risk of illiquidity in the models, but he warns that the equations obtained from the model do not have a single and clean solution. Illiquidity, by its very definition, is unpredictable: no mathematical model can predict when buyers in the market will decide that it is not worth investing any amount of money in a certain financial instrument because of the risk involved. In order for such behavior to be reflected in the model, it must allow for a variety of solutions, and the choice between them may be problematic. "The models I work on can be useful in assessing the risk of illiquidity, but they are far from perfect," says Jaro.
Unfortunately, ignoring the illiquidity risk was not the only serious problem. Financial risk forecasting models were designed to focus on the risk faced by one particular institution.
This is always considered reasonable because financial institutions only want to deal with the risk they themselves are exposed to, and the regulatory bodies tend to act based on the assumption that if the risk posed to each and every one of these institutions is low, the system as a whole can be considered safe. But that turned out to be a weak assumption, says Rama Kunt, who heads Columbia University's Center for Financial Engineering. According to Kont, in a system where there are interdependencies between many factors, the systemic risk as a whole may be high, even if each of the factors by itself has a low risk of failure.
Imagine, says Conte, a group of 30 people marching side by side in an open field with everyone's arms resting on the shoulders of their friends: each of them may not be expected to stumble, but there is a reasonable chance that someone in the group will stumble and in this case, they may fall along with others How many of his friends. This is exactly the situation in which financial institutions are found, according to Conte. "Until 2008 and even during it, the regulators, when they came to assess risks, did not pay any attention to the connections between these banks, he says. "They should have, at the very least, noticed that everyone invested a huge amount of capital in the subprime mortgage market."
The disaster map
The power generation industry faces a similar problem, says Conte. The chances of failure in a single power plant are minimal, but from time to time a failure occurs in some power plant, which may cause an overload in other power plants in the network, a situation that has the risk of a large-scale shutdown of the electricity supply, such as the large power outages that occurred in the USA in 1965, 1977 and 2003. In order to reduce the risk of this type of system failure, electricity supply companies conduct tests known as "N-1 testing": running scenarios in which a single power plant failure is simulated in order to predict what its effect will be on the entire network. However, as Kont says, the power generation industry has a considerable advantage, since it knows how all the power plants in the system are interconnected.
The financial system, however, is a black box. "In the current state of affairs, no one knows what the financial system looks like," says Conte. "We don't know who is negotiating with whom, regarding what and at what price. This means that we cannot predict the consequences of the collapse of an investment bank such as Lehman Brothers on other banks. In 2008, the regulators had 48 hours to come up with a guess."
The obvious solution is to map all these connections. Kont is among the lobbyists working to enforce on financial institutions the obligation to report to a central government body to collect data on all the transactions they manage, not only at the local national level, but also at the international level, since today money flows freely from country to country. However, banks are naturally reluctant to report this data. If a company discloses to the general public details of a large-scale investment that is in the discussion and formation stages, this may inspire others to imitate it and buy the same, thus causing an increase in prices, while information regarding a large-scale general sale may indicate financial problems and cause investors to withdraw their money. According to Kont, it is possible to address the problem of confidentiality and ensure that the reports sent to the data collection agency are not distributed to other parties.
"For years, governments have been sharing classified data regarding nuclear capabilities with international agencies," notes Cont, "and financial data is no more sensitive than that." In fact, the Dodd-Frank reform that was approved and became an official law in the USA in 2010 allows for the establishment of a "government office for financial research", which may basically be used as an agency to collect data from American financial institutions. However, it is still unclear whether any agency will be able to collect all the data necessary to draw a detailed up-to-date map of the global financial system, so systemic risks can still go unnoticed just as they did in 2007.
Even if the regulators have enough data, the models at our disposal are not sophisticated enough to handle them. According to Professor Darrell Duffy of Stanford University, the existing models are probabilistic models that do not make any assumptions about the future, and instead provide a list of the chances of default in all kinds of situations among countless possible future situations. It goes without saying that a reliable determination of such chances requires not only the collection of mountains of data, but also a thorough understanding of all the different forces that play a role in the arena, complicated mathematics and tremendous computing power. And that's just for a single bank. According to Duffy, the very idea of applying these terrifying requirements to the financial system as a whole borders on the absurd.
Duffy makes an alternative proposal: a stress test or, simply, a detailed description of some well-defined future scenarios that could expose a banking institution to extraordinary risks. Identifying the risk of insolvency under the conditions of a limited scenario presents us with a simpler problem. For example, if we are trying to find out for ourselves what the risk is that we will not be able to meet the repayment of her mortgage payments at some time in the future, we can simplify the problem if instead of calculating how we will manage in several possible future events, or in all of them, we try to guess how we can overcome a 10% cut in our salary. Banks can choose scenarios that include a sharp drop in the stock market, default on mortgages, skyrocketing interest rates, and so on. In these scenarios, a situation where one or more financial institutions are unable to repay their debts will also be taken into account, in order to check how such a situation of insolvency may affect the bank conducting the test. "The idea is to simulate serious shocks in the bank's investment portfolio and see what the bank's performance will be from there on," says Duffy. "It is not so important what the probability of any particular scenario materializing is; In any case, the scenario provides us with a lot of information for the purpose of locating weak points."
Duffy recommends asking the banks to respond to about 10 different scenarios that will be carefully selected, and that each of them will include the possibility of insolvency of each of 10 different banks. According to him, if 10 banks conduct such an examination, we will get a 10x10x10 matrix, which can give the regulators a pretty good idea of where the systemic risks are.
If a few major banks had been specifically asked a few years ago, say in 2006, to assess how their investment portfolios would be affected by a wave of mortgage defaults and the collapse of two huge financial institutions, it is quite possible that regulators would have had all the information necessary to prompt the financial system to take An appropriate action for a smooth escape from the dangerous situation in which she found herself. The disadvantage of this approach, Duffy admits, is that a stress test can only examine a very small number of the countless possible scenarios that the financial system may face. It is impossible to require a bank to produce risk assessments for thousands of different scenarios that include the insolvency of hundreds of different banks. This means that even if the test using scenarios shows that the system is relatively stable and capable of withstanding the tested shocks, it is still possible for the system to collapse if one of the countless scenarios not included in the test is realized.
The mathematical golden path
Another problem involved in building complex models is that at a certain point their complexity becomes an obstacle. Paul Wilmot, former manager of a hedge fund and now a researcher dealing with applied mathematics, says that often, the builders of the models cause their creations to get stuck in the mire of dozens of mathematical expressions loaded with various variables and parameters, each of which is essentially accompanied by a new possibility for error, so that the final result is far from accurate . Wilmot recommends that the model builders try to find the "mathematical golden path" as he calls it, that is, the point where the model has enough expressions to allow a reasonable approximation of reality, and yet it is still simple enough so that we can fully understand all its operations and limitations. Not many model builders manage to achieve such a balance, Wilmot says.
It is safe to bet that the reliability problem of financial risk assessment models will not be solved for many years to come. So what can we do about it? The only real option before us is not to trust the models, even if theoretically, the equations look really good. But such thinking contradicts the tenets of Wall Street's belief. "There was never any incentive to question the models, because the people in control make a fortune from using them," Jaro says. "Until the outbreak of the crisis, everyone thought that the models worked well. And now they are trusted again." The models and data are expected to improve, he states, but not sufficiently to warrant too much confidence in the results.
If the regulators had heeded these warnings, they would have forced the banks to hold more cash and exercise greater caution in their investments. The price of this caution, which does not exceed the reasonable limit, will be a less efficient system, Avalanda points out, or, in other words, investors will get less rich from it, on average. Banks' profits will decrease and they will have less money available to provide loans. It will be a little harder for all of us to manage in this situation, but it will also be less likely that we will jump headlong into another crisis with our eyes closed. Everything has a price.
David H. Freedman has been writing about science, business and technology for 30 years. His latest book, Wrong!, explores the forces that drive scientists and other senior experts to mislead us.
About the author
David H. Freedman has been writing about science, business and technology for 30 years. His latest book, Wrong!, explores the forces that drive scientists and other senior experts to mislead us.
And more on the subject
The science of economic bubbles and their expiration. By Gary Stix, Scientific American Israel, October-November 2009, pages 54-61.
The Failure of Risk Management: Why It's Broken and How to Fix It. Douglas W. Hubbard. Wiley, 2009.
Too Big to Fail: The Inside Story of How Wall Street and Washington Fought to Save the Financial System - and Themselves. Andrew Ross Sorkin. Penguin, 2010.
Risk Management Models: Construction, Testing, Usage. Robert A. Jarrow in Journal of Derivatives, Summer 2011, Vol. 18, no. 4, pages 89–98; Summer 2011.
6 תגובות
The amount of text and the strange comparisons the author of the article chose to explain his opinion is an expression of the real problem: too little logic in investment management and too much philosophy. Bottom line, the capitalists tried as always to maximize the investment and the regulation that was supposed to prevent exactly such scenarios collapsed.
I recommend reading studies on the social and class system
In a group or herd of various types of animals, wolf monkeys ……….
In principle, in terms of positions and relationships, the exploiter is exploited by the strong and the weak
Ruler ruled, there it is just like with the human species.
All that is left for the weak, my anonymous friend, is to protest, to get angry, to curse, to cry
But again if you check there the weak animals do the same thing.
Note that when there is a revolution and out of the revolutionaries leaders arise
new and after a period of time the same situation returns again exploiting the exploited.
poor rich strong weak
Researchers will say that tears from an evolutionary point of view were created only in the eyes
of the creatures of the lower class in order to blur the landscape of reality.
The problem is actually the stinking neoliberal system that allows these charlatans to gamble with public money without being held accountable.
The emotion, it is the one that controls, the one that ultimately decides, it works in a hidden way that cannot be defined or predicted.
Everything we do, we take completely random decisions and results from the past, and build theories on them,
Suddenly, in a very strange way contrary to a logical solid theory, an economic crisis breaks out, war, epidemic, famine....
And again research, conclusions, proposals, theories, budgets... circles that repeat themselves endlessly..
The difference between them is only the size of the radius.
There are professions whose main goal is to complicate everything, as much as possible, so that they don't understand anything.
with the aim of raking in as much profit as possible.
There are those ignorant people who work hard for pennies
And there are those who print money
Since there are too many workers in the profession, everything has become even more complicated, to the point that they themselves do not understand what is happening, and thus the capital market has become prone to disasters.
Too many people are stirring the plate.
The only model that exists is that the rich get exponentially richer.
The proof is very simple, and is a result of the method working on percentages.